A computationally efficient hybrid model for simulating the additive manufacturing process of metals
Abstract
Additive manufacturing (AM) suffers from residual stress formation and part distortion. One way to improve AM is to use optimal process parameters that yield residual stress distribution that is less detrimental. A finite element analysis (FEA) based model can be used as a design tool to determine optimal process parameters. Being a design tool, such a model should not only predict the residual stress formation and part distortion accurately but should be capable of performing the simulation in a reasonable time as well. However, the traditional FEA models are either accurate but computationally very expensive as in the case of process-based models that captures the evolution of the temperature and stress/strain field or very fast but often inaccurate as in the case of inherent strain based (IST) based models which comprise a series of quasi-static stress-analyses. In this study, we demonstrate that a model that is both accurate and computationally less expensive can be developed by adopting a hybrid approach in which the spatial and temporal resolution of the field solutions over different regions of the part are selectively controlled depending on the expected levels of stress gradients in these regions. The new model, called Additive FEA Hybrid (AFEA-H) model, uses a previously reported Additive FEA (AFEA) framework [1] to combine a process-based model that has a high spatial and temporal resolution with an IST based model that has a low resolution. Numerical simulations performed for a few example problems demonstrate that the AFEA-H method can enhance the computational efficiency substantially without compromising solution accuracy.
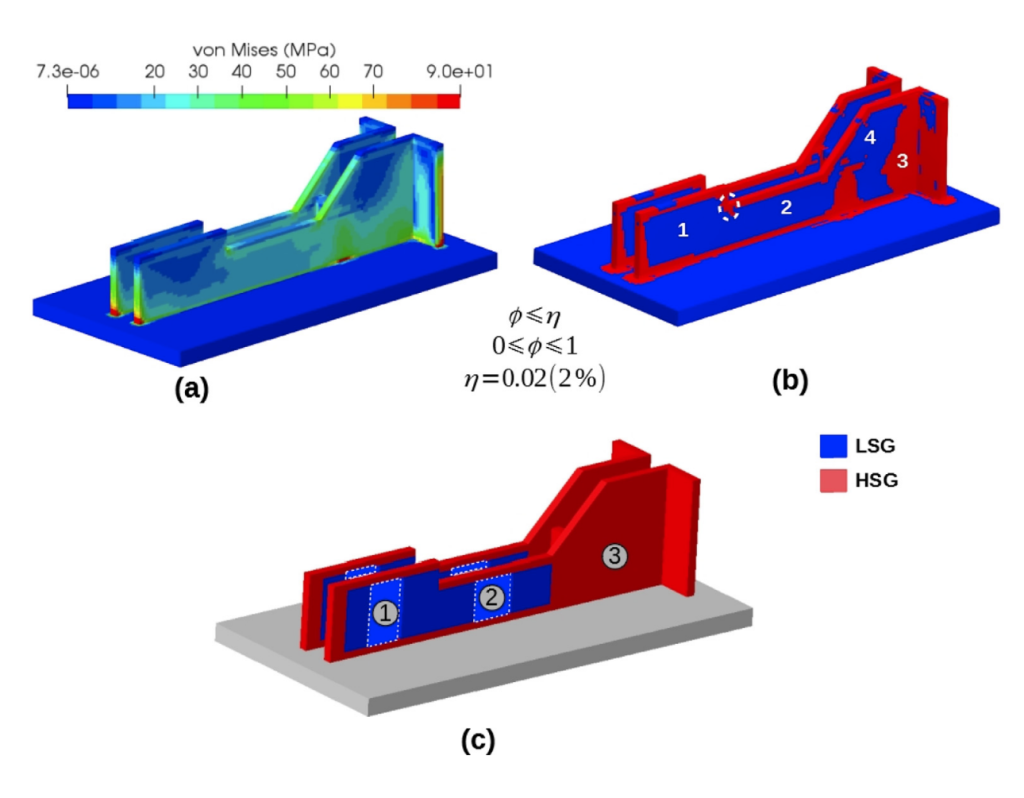
gradient (c) Divided regions as LSG and HSG
regions for AFEA-H simulation
Characterization of microscopic deformation of materials using deep learning algorithms
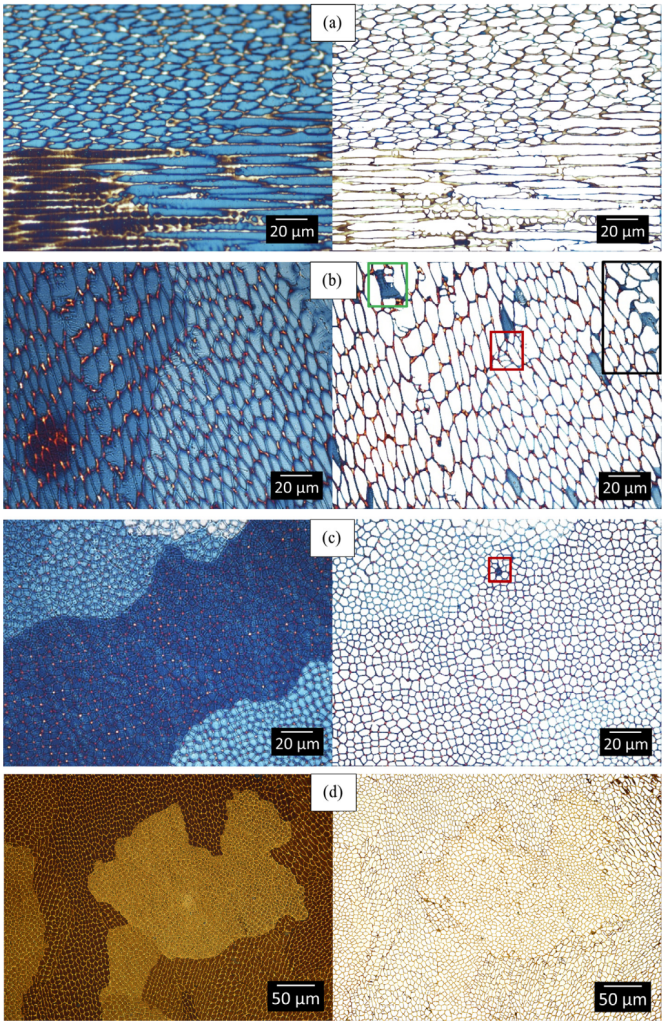
Abstract
Microstructure-informed design approach is set to revolutionize the design of metals and alloy components for aerospace applications. In this approach, a designer utilizes the influence of individual microstructural features on microscopic deformation to yield desirable macroscopic properties. Therefore, the development of advanced experimental capabilities that enable detailed characterization of microscopic deformation of material test specimens is critical to realize this paradigm shift in practice. However, extracting the complex characteristics of microscopic deformation hidden in raw image data is quite challenging. In this article, we propose an automated data extraction and analysis method based on instance segmentation and tracking of microstructural features using deep learning (DL) and image processing algorithms. The method consists of a trained mask Region-based Convolutional Neural Network
(mask R-CNN) DL model combined with a regional instance segmentation approach for the instance segmentation of features, an intersection over union based multi-object tracking method to track segmented instances as they deform, and kinematics models to extract the material characteristics from the geometrical data of the deforming instances. The method is then validated by characterizing the microscopic deformation of an additively manufactured 316L stainless steel coupon specimen under quasi-static tensile loading. Our study presents a general framework for advancing deep learning algorithms to solve
complex problems in the field of experimental mechanics.
Influence of cellular subgrain feature on mechanical deformation and properties of directed energy deposited stainless steel 316 L
Abstract
The fundamental deformation mechanism responsible for the exceptional combination of strength and ductility exhibited by SS 316 L material manufactured using directed energy deposition (DED) based additive manufacturing (AM) technique was studied. First, the stress-strain behaviors under uniaxial tensile testing of specimens containing different intensities of cellular subgrain feature were obtained. The stress-strain behaviors showed large differences with different intensities. Then, the microscopic deformation under in-situ tensile testing was characterized. At intragranular length scale, results revealed an extensive formation of a heterogeneous surface texture with a characteristic length scale and morphology consistent with the geometry of the cellular subgrain feature. The results also revealed that the high strain hardening rate regime of the macroscopic stress-strain behavior is primarily driven by deformation slipping, not deformation twinning. Finally, the evolution of the slip bands under uniaxial tensile testing was characterized using scanning electron microscopy (SEM) and atomic force microscopy (AFM). Results revealed a barrier effect of cellular subgrain feature on slip band evolution. For a given macroscopic strain the width, depth, and spacing of slip band formation varied with the intensity of the cellular subgrain feature. The heterogeneous texture formation and barrier effect on slip band evolution observed in this study were clear evidence of the extensive influence of cellular subgrain feature on microscopic deformation and mechanical properties. Additionally, these remarkable observations revealed that dynamic slip band refinement induced by cellular subgrain feature is the fundamental mechanism that facilitates the exceptional combination of strength and ductility of DED manufactured SS 316 L.
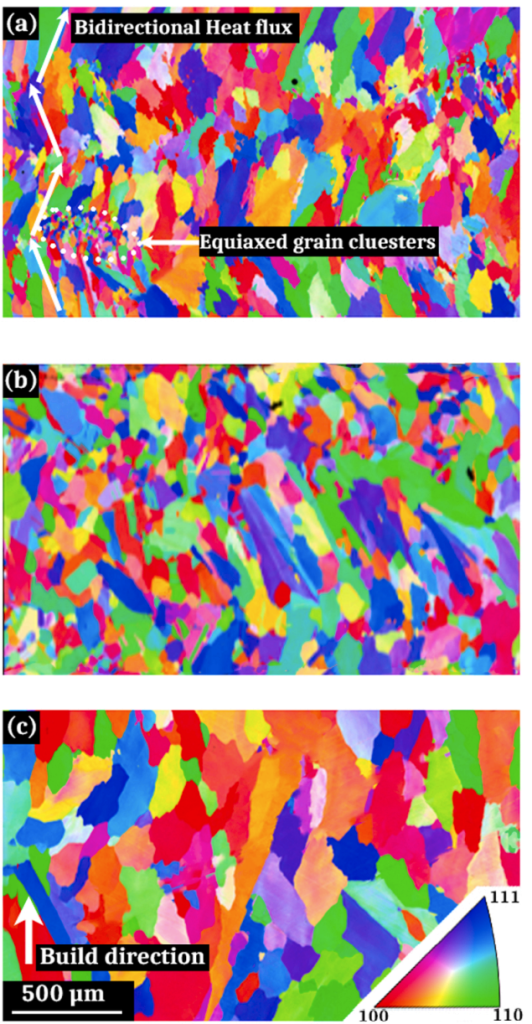